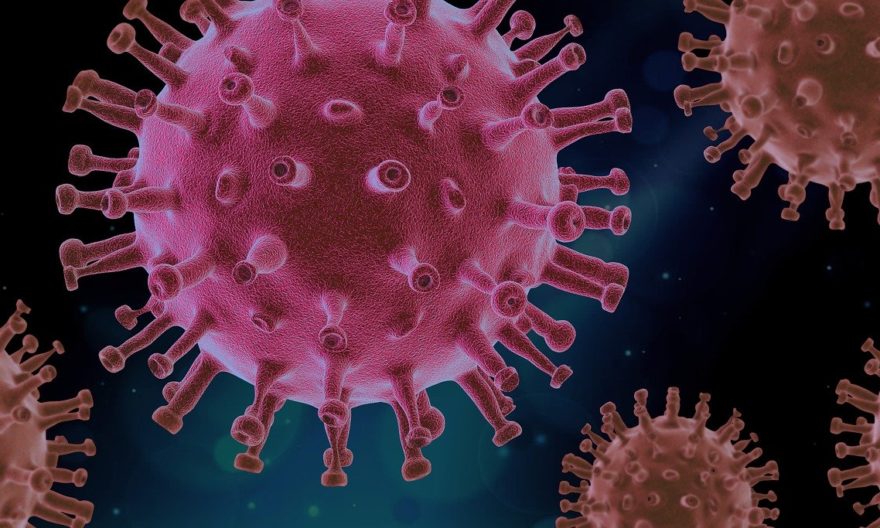
Overview
Pandemic is an extended version of an epidemic, which spreads among people beyond a geographical area. As time passes the number of pandemic disease increases and now we have reached till the most infectious and threatful orthocoronavirinae from the family coronaviridae. The first case of COrona VIrus Disease 2019 (COVID-19) was detected in Wuhan, a city in China around 17 November 2019. After 7 to 14 days of vulnerability, there will be symptoms of high fever, cough and also shortness in breathing. In the span of 3 months, COVID-19 infected more than 3 lacs of people around the world. Here, we will deep dive into the prediction and spreading of pandemic diseases with Machine Learning models and classifiers.
In Machine Learning, many models are built for the prediction of the epidemic as well as pandemic diseases. Here we are discussing NaiveBayes, RandomForest and Adaboost classifiers. Apart from them, there are SVM, XG-Boost, Gradient Boost and much more.
We can also find the spreading of this pandemic disease to a larger extent, here we will go through the basic compartmental model used to find the disease spread among the people. Machine learning makes our model learn the dataset and make the most accurate prediction of the disease about the future. Machine learning also aims to provide the spread of disease among the individuals and thus help us in taking necessary precautions for the cause.
What is pandemic Disease?
Pandemic is a type of epidemic disease but is extended to all over the globe. Pandemic flu was the first pandemic disease. Thereafter came Smallpox, Monkeypox, Nipah virus, SARS, HIV/AIDS, and now we are in the outbreak of the Novel Coronavirus. The meaning of pandemic is widespread or prevalent which means it can spread all over the country or world. And It can be an outbreak of all diseases.
How will we predict Pandemic Disease?
Before building our model or classifier we have to go under these stages for prediction of pandemic disease.
- Retrieval of the dataset: Collection data set from websites or tweet datasets
- Preprocessing the dataset: This is the most important step before building the model. Here we clean the dataset by transforming raw facts into an informative format.
- Feature Extraction: This is the process of removing noisy or unwanted columns from our dataset
- Train-Test split: here we split our dataset into training and testing data which can be in proportion of 70% and 30% respectively.
Types of Classifiers
After undergoing the stages which are mentioned above, we are ready to build our model. Machine learning algorithms that give a categorical or binary value as output is called classifiers and process is called classification. The classification algorithm classifies a group of values in the training set into clusters and predict the result.
Naive Bayes Classifier
Here we mention Naive Bayes also known as a probabilistic classifier, which is one the simplest and basic classifier.let the features vary from x0 to xn and our classes vary from c0 to cn. The model checks the probability of feature occurring in each class and predicts the most likely occurring class. Naive Bayes uses the famous Bayesian rule and performs the prediction.
As the name says its naive or has a lack of experience, hence gives a poor accuracy rate compared to the rest of the classifiers. Naive Bayes gives a better result for multiple classes as well as for text classification.
Random Forest Classifier
Random Forest is an ensemble classifier which means it consists of a group of algorithms or classifiers. It develops random numbers of decision trees from the training dataset and uses the majority voting method to assign votes for the decision tree and predicts the final class which has the highest vote. This gives a better accuracy rate when there is a large dataset and when there are more missing values. we can use CORONA VIRUS (COVID-19) TWEETS DATASET that contains the live feed for COVID-19 virus-related tweets.
Adaboost Classifier
This is a boosting classifier which means improved or better. As its name says it is a better classifier compared to naive Bayes. It uses a majority voting method. It is also an ensemble classifier like the random forest classifier. They assign weightage after training each classifier and also based on the accuracy of each classifier.
The more accurate classifier has the highest vote and predicts the outcome depending on the highest vote. This classifier is better than a Random Forest classifier because it gives weak classifier the final decision, hence give a better accuracy rate than random forest classifier.
These models help us to predict the outbreak of epidemic or pandemic diseases like coronavirus. Next from this model we try getting the predictions and evaluate the results.
Predicting a pandemic disease such as corona, flu pandemic, etc can help individuals in a particular place to take safety measures against the pandemic outbreak.
How will we find the spread of a pandemic outbreak?
The spread of this pandemic coronavirus is so fast and rapidly. It can be passed through air, water or through any sources. Here we use compartmental models to identify the spread.
In these models, there are 3 main compartments:
- susceptible are those individuals who are likely to be infected by the disease.
- Infectious is the number of infected individuals.
- Recovery are those individuals who have retained their immunity.
There are mainly 3 types of compartmental models SIR, SIS, and SI model.
Image Credit: Nature
In the case of the SI model, individuals from susceptible states move to the infected state and remain infected throughout. Corona is not a disease where individuals remain infected throughout so we don’t use the SI model for identifying the spread of the disease.
Fig2: Here beta is the transmission rate
SIS is a model similar to the SI model where an individual does not move to recovery state but move to the susceptible state, hence this model also cannot be used for the spread of coronavirus
Fig3: Beta is the transmission rate and gamma the recovery rate
In the SIR model, individuals from susceptible states move to the infected and from their move to the recovery state. Also, individuals affected with a disease or in the infected state, they move to recovery state or in other words disease can be recovered by the individuals and can retain their immunity. Coronavirus is a disease that can be recovered, hence we can use the SIR model to predict or identify the spread of disease among individuals.
Conclusion
Getting nervous is not a solution rather takes and spreads awareness among society. Prediction of this virus makes people aware of the disease and can take precaution measures and can help the country to fight against the disease. Spreading models helps the individual understand the spread of disease. We have heard the saying “prevention better than cure”, it is better to take precaution for the disease rather than looking for cures for the disease. These prediction and spreading models help the people to understand the rates and transmission of disease and can take action at the earliest.
Read Next: Hackers steal sensitive information exploiting COVID-19 outbreak
go corona go
Excellent information to understand this deadly virus
Well written….for being the first attempt
Very informative write up. This could give some guidelines for controlling this virus.
Nice article
Stay together….
“Prevention is better than cure” the old saying yet again proves that it is true. With these type of algorithms that could predict pandemics it would be of great help to health workers,scientific communities as well as to the government bodies to make a better plan to prevent such outbreaks.
Good information.
Informative
good one
Amazing Write up..
Well written…
Good. You should have discussed precautionary measures as well
Well said
Excellent write up.
Stay home and be safe everyone
Good information and well written
Quite impressed with this article of yours dear. Keep it up.
Well written pooja..
Stay home and fight together
Well written
Well written and informative…
Excellent work….very informative and well written…All the best dear
Thank you for the information.
Good one.. highly informative
Very informative article
Amazing Write up..
Stay at home!! Lets fight corona together!
Good one..
A lot of detailed work has been done to put up this article. Would definitely help mankind if it works out as it’s written.
It’s Well Explained and Social Distance is must for any Viral Infections
Jst break the chain…
Go Corona
Informative
Good information
Stay safe, stay healthy, break the chain!
#goCoronaGo!
Well documented and very informative.. well done
Interesting. Hope Science will save humanity from this challenge as well. Best Wishes
Good information and well written
Good information
Very well articulated piece of work. Fact filled, yet explained in simple terms to benefit people belonging to grass root level, as far as this topic is concerned.
One suggestion. The susceptible, infections and recovered population are represented with circles of equal diameter, which actually is not in actual practice. It would have been more realistic if the disease transmission and its recovery rates are closely monitored, the data captured out of it and circles resized to show the representative fraction.
Good attempt at a rate of 9 out of 10.
Well said. At this time we need precautions not just panic.
Good information. Well written.
Let’s stay home and save our society.
Let us all be Channels of blessing……..
Well said. At this time we need precautions not just panic
Poli sanam… Nice article
Nice one!
More information
More safety
Nice article and highly informative one…..
Knowledge information , you have done well, keep it up. Now we have only one solution as you said above ‘prevention is better than cure’. Obey all rules from health department and be safe. Pray for all.
It’s very informative, but our public should take seriousness of this virus as it can be prevented with several remedies in full awareness of this virus. Prevention is better than cure.
Very Informative ✨
Well said
thats great
Good information.
Well written
Well documented pooja and good work…
Worth a read.
Very informative and well explained
Well written
Healthy information,apt in driving attention of common people towards the growing disaster
Well said !
Go corona
Well written
Good work …. BREAK THE CHAIN !
United we loose…
Divided we win…
Well written
Great work Poojaa
Well written, let’s fight against the disease
Well explained…
Well written article and really very informative. Keep it up…. Suresh
Nice work on Corona virus.
Well written!keep going
Actual facts. Very informative. Keep it up.
Really informative and well written… Keep going and All the best… suresh
Well written….lets break the chain..
Well said…lets break the chain..
Well written✌
Great knowledge shared Pooja Awaiting many more informative blogs!
Nice Article…Very specific and Informative
Very informative
Nice work
Very informative….well said
Very informative
Good work
When some one searches for his vital thing, so he/she desires to be
available that in detail, so that thing is maintained over here.